Why you should make your AI product harder to use: The Egg Effect
A counterintuitive insight from the 1950s cake industry
➡️You could sponsor this post: msg sponsoring@productea.io and we’ll send you our media kit
Here's a puzzle from the 1950s: How do you sell more cake mix?
Answer: Make the cake harder to bake.
When instant cake mixes hit the market, manufacturers discovered something strange. Sales were sluggish. Many housewives—their target customers—resisted these convenient new products.
The problem? Food historian Laura Shapiro says that the cake mixes made baking too easy. It was too convenient! There was no pride in the process, no sense of achievement.
The solution came from an unlikely place: an egg.
Manufacturers modified their recipes to require adding a fresh egg to the mix. Acclaimed food historian Laura Shapiro says this small change transformed everything. Sales for General Mills and Duncan Hines soared.
By making the process slightly more demanding, manufacturers made the product more appealing. But why did making something harder drive enjoyment and usage?
The science of user investment
Behavioral scientists Michael Norton, Daniel Mochon, and Dan Ariely later demonstrated this phenomenon through a series of experiments involving IKEA furniture, origami, and Legos.
In the origami experiment, participants built their own origami figures by following detailed instructions. Then these builders were asked (along with people who didn’t build anything) how much they would pay for their creation.
What happened? The builders offered nearly 5x more for their own creations (around $0.23) compared to non-builders (around $0.05) who were buying someone else’s creation.
Even more striking: builders valued their amateur creations almost as much as expert-made origami.
It turns out: people value things more when they help create them. In these experiments, this finding held true whether the items were practical, like furniture, or recreational, like origami.
What can AI product designers learn from eggs and origami?
This psychological insight has implications for AI product design today. We may need to let customers add some eggs and build their origami.
Just as the egg created a sense of ownership in baking, user input in AI systems creates a similar psychological investment.
Just as origami makers overvalued their own creations despite their imperfections, users might value AI outputs more if they feel they've contributed to creating them, even if the contribution is relatively small.
In an experiment in Germany, researchers found that people valued AI-generated images significantly more highly when they actively helped refine them, compared to receiving completed AI outputs. The effect was particularly strong when participants could iterate on elements like composition and style–they reported both higher satisfaction with the final images and greater willingness to use them in professional contexts.
Yet, at Irrational Labs, we see most companies moving in the opposite direction.
They promise magical solutions that require very little human input: "Just write a quick prompt and let AI do the work!" This approach fundamentally misunderstands human psychology.
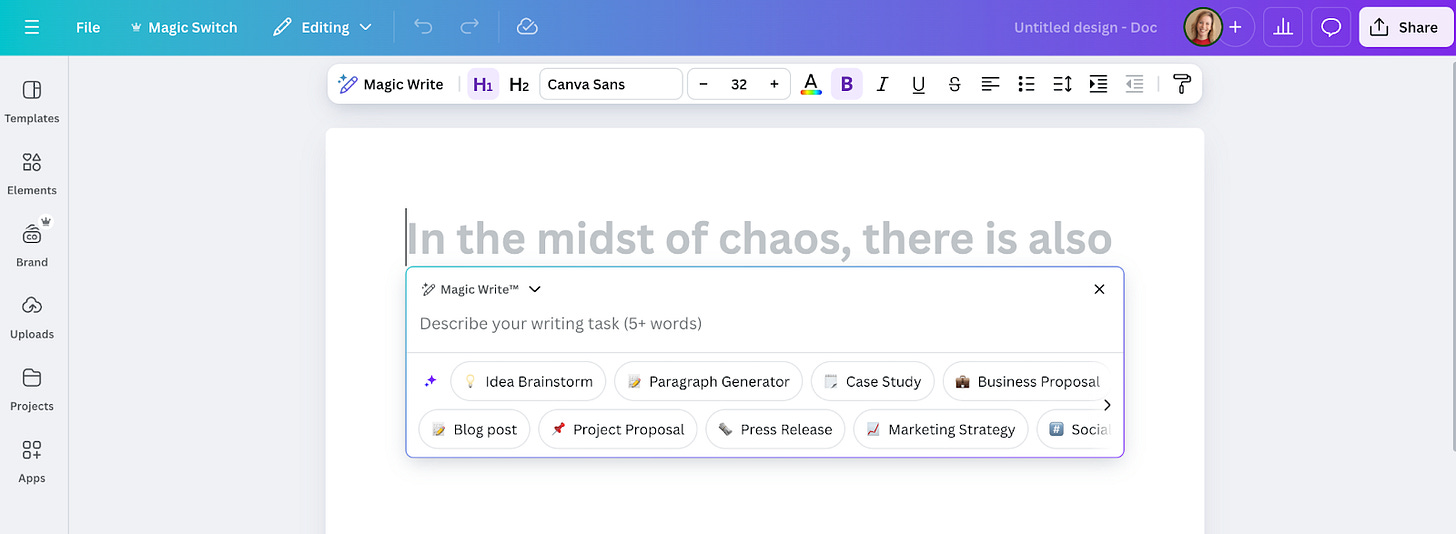
Consider real-world parallels. Imagine if these people never involved you in the process!
A matchmaker who never asks about your preferences or past relationships
A financial advisor who invests your money without understanding your goals
A writing coach who never reads your work
We would instinctively distrust these services. Why? Because meaningful outcomes require meaningful input.
The same principle applies to AI.
When users invest effort in training, customizing, or directing AI systems, they're more likely to trust the results and value the output–even if the technical quality remains unchanged.
Building better AI products by involving the user
The challenge for AI products is finding the sweet spot–requiring enough user involvement to create investment and ownership, but not so much that it becomes burdensome. Here are some ways this could work:
Writing assistants:
Have users share examples of their writing before generating
Let them pick which writing styles they like best (thumbs up/thumbs down, like Netflix)Image creation:
Ask users about their style preferences and tastes/vibe (like we do with human designers)
Give multiple options and assume the first round of edits needs their refinement (and that’s a good thing)Personal assistant
Ask users about their life and preferences (beyond what industry they are in and size of company)
Get them to provide as much historical info as possible (yes, sync your email and drive)
This approach is particularly crucial in domains where success criteria are subjective. Research by Christoph Fuchs shows that people consistently rate their own ideas more highly than ideas attributed to others–highlighting our tendency to value what we help create.
So even if your AI is better than what a user would do by themselves (and it likely is!), that doesn’t guarantee that the user will appreciate your output better than their own.
Real-world examples
Several companies are already leveraging this principle effectively:
Enterprise SaaS: Salesforce requires new enterprise customers to invest time in discovery and setup, customizing workflows to their specific needs. This upfront investment can increase initial adoption and renewal rates.
Consumer products: Function of Beauty has customers do an extensive hair quiz to customize their products. This involvement justifies premium pricing ($94 for conditioner?!) and strengthens brand loyalty.
Finance: Betterment and Wealthfront have succeeded by flipping the traditional "robo-advisor" model. Users have to complete an extensive personal financial assessment. Customers set specific life goals, connect existing accounts, choose investment themes, and adjust allocations within guardrails.
Another great example in the enterprise SaaS space is Glean, an enterprise AI search tool.
Glean succeeds by understanding a basic truth: companies want to teach the AI about their own data and how they work. Yes, Glean has good security and privacy controls, which is table stakes for enterprise clients. But what really matters is that companies get to put in the work to train the system on their systems.
Like adding an egg to cake mix, this effort pays off. The system works better, and companies trust it more because they helped build it.
The power of user participation
The lesson is clear: Don't try to eliminate user effort entirely. Instead, channel it productively.
Give your users eggs to crack. Let them fold their origami. When people invest in the process, they invest in the outcome.
Product teams should ask:
Where can users meaningfully contribute their expertise?
How can we make user input feel valuable rather than burdensome?
What’s our equivalent of the cake mix egg?
Want better AI product adoption? Get the user to put in some work.
Great post.
This reminded me of this one from Noahpinion last year (this is the quote I always remember): https://open.substack.com/pub/noahpinion/p/what-if-everyone-is-wrong-about-what?r=2ilv0&selection=e2652e9c-2165-470e-8d26-9ffcffde9452&utm_campaign=post-share-selection&utm_medium=web
> When users invest effort in training, customizing, or directing AI systems, they're more likely to trust the results and value the output–even if the technical quality remains unchanged.
In my view, we're not yet at the point where the quality of the output from an AI-only service can match one that is AI + human. So, from a user psychology perspective (as you mention in the post) and a functional one, it makes so much more sense to keep the "human in the loop".
Love this. It's just another type of friction and less friction is not always better.